New classification methods for data with incomplete observability
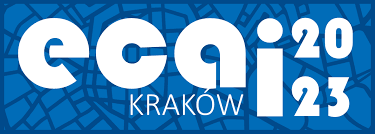
The Statistical Analysis and Modeling Group of IPI PAN achieved significant results regarding two new machine learning methods for positive-unlabeld data in an important case when their availability depends on the characteristics of the examined entities.
The work "Double logistic regression approach to biased positive-unlabeled data" concerns inference methods in a classification problem with incomplete observability called positive-unlabeled data, in which only part of the observations from the positive class are labeled, while the rest are not labeled. This type of data is often found in biology, medicine, recommender systems, and the problem of web page tagging. In applications, the situation considered in this work is particularly important when labeling depends on the characteristics of the object. The concept uses a parametric approach to the problem in which both the posterior probability of the positive class and the labeling propensity score function are modeled using a logistic model. The paper resolves the issue of the identifiability of the parameters of such a model, proposes a method for their estimation and shows that it is effective in practice.
In the paper "One-class classification approach to variational learning from biased positive unlabelled data" a different approach to the same problem was considered based on minimizing empirical risk, and not requiring explicit modeling of the labeling propensity score function. The method is based on the use of learning variational autoencoders combined with outlier detection methods, which allows us to identify observations from the group of unlabeled observations that are most likely to come from the positive class. The implemented method yields a significant improvement in the performance of the resulting classifiers compared to previously proposed methods, especially for low probabilities of labeling.
Both methods were presented at the European Conference on Artificial Intelligence, ECAI 2023 and published in its materials:
- K. Furmańczyk, J. Mielniczuk, W. Rejchel, P. Teisseyre (2023), Double logistic regression approach to biased positive-unlabeled data, Proceedings of the European Conference on Artificial Intelligence ECAI’23,
- Wawrzeńczyk, J. Mielniczuk (2023), One-class classification approach to variational learning from biased positive unlabelled data, Proceedings of the European Conference on Artificial Intelligence ECAI’23.